Currently, in all my classes, I use design of experiments (DOE) to help us better understand the factors that are most important in insect rearing systems. I originally became aware of the applications of DOE through a criticism of my statements about using one factor at a time for diet development in my book on insect diets (Cohen 2004). The criticism came from Dr. Steve Lapointe et al. (Lapointe, S. L., T. J. Evens, and R. P. Niedz. 2008. Insect diets as mixtures: Optimization for a polyphagous weevil. Journal of Insect Physiology. 54: 1157-1167.) I was initially dismayed at the criticism of my conventional (and outdated) thinking that we could only use one variable (one factor) at a time in a good scientific experiment. Once I got over the ego-deflation of having a blatantly narrow statement in my book, I set-out to learn how multiple factor experiments could be far more telling about the nature of our diets and other rearing system components.
As a start in my new-found application of DOE, I included this more sophisticated approach in the 2nd edition of my Insect Diets: Science and Technology, but I still had a steep learning curve. After years of effort to learn how to use the JMP approaches to DOE, I have reached a point where I routinely use various DOE tools (response surface designs, full-factorial designs, mixture designs, and Taguchi designs–all of which have their own specific applications). I have included extensive tutorials on using JMP’s system of DOE-based programs in my newest book, Design, Operation, and Control of Insect Rearing Systems (2021, CRC Press).
I want to make a special point about using JMP’s DOE: I am a physiologist, NOT A STATISTICIAN. Despite my trying to learn how to apply DOE, once I learned how powerful it could be in helping me understand the science behind insect rearing system dynamics, it was still a steep learning curve for me. I realize that most of my fellow rearing professionals are NOT statisticians either, and that is why I believe the JMP system’s DOE is so helpful. It allows people like me to ease their way into the very powerful statistical programs that can be so helpful in understanding what is going on in our rearing systems. The following mini-case study helps illustrate this point:
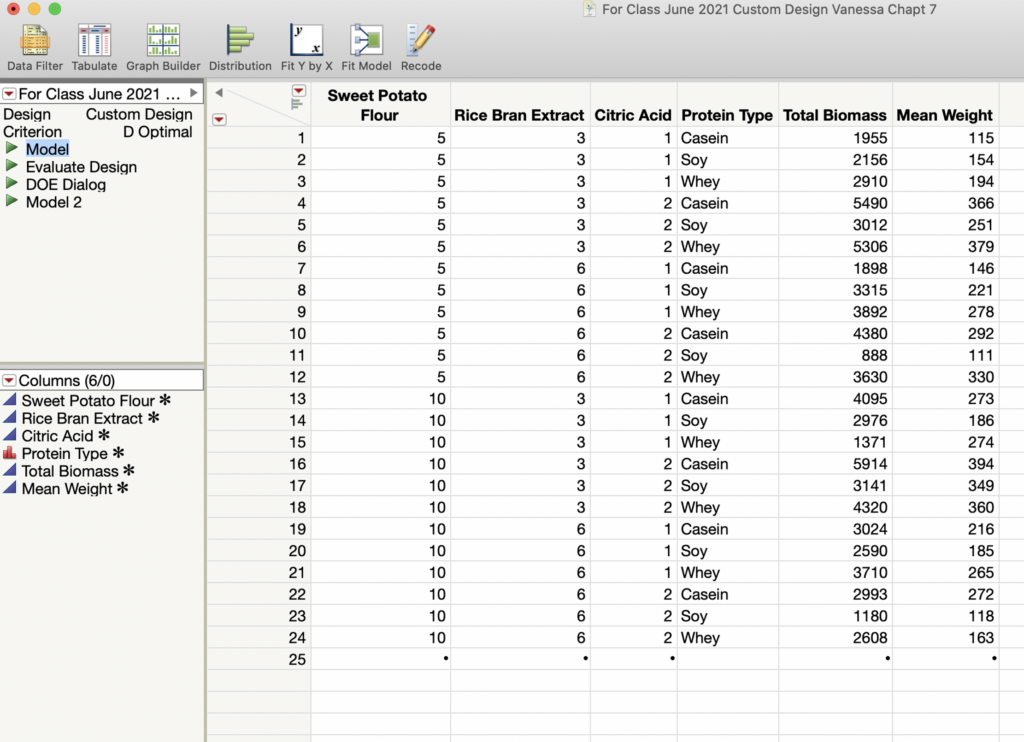
In Figure 1, I present a test that I did with painted lady butterflies (Vanessa cardui). In this test, I was asking the questions about the efficacy of sweet potato flour, rice bran extract, citric acid, and three types of protein (casein, soy, and dairy whey). The table in Figure 1 shows that I used two levels of sweet potato flour, two of rice bran extract, and two of citric acid while I provided casein, soy, or whey proteins. This full-factorial design required that I make up and evaluate 24 different diets (all of which had the same proportions of the other diet components not shown here). In the last two columns (on the right), the responses of total biomass per rearing unit and mean weight of individuals are presented. Feeding these data into the JMP system resulted in a complete statistical analysis of the experiment, including this convenient summary of the outcomes:
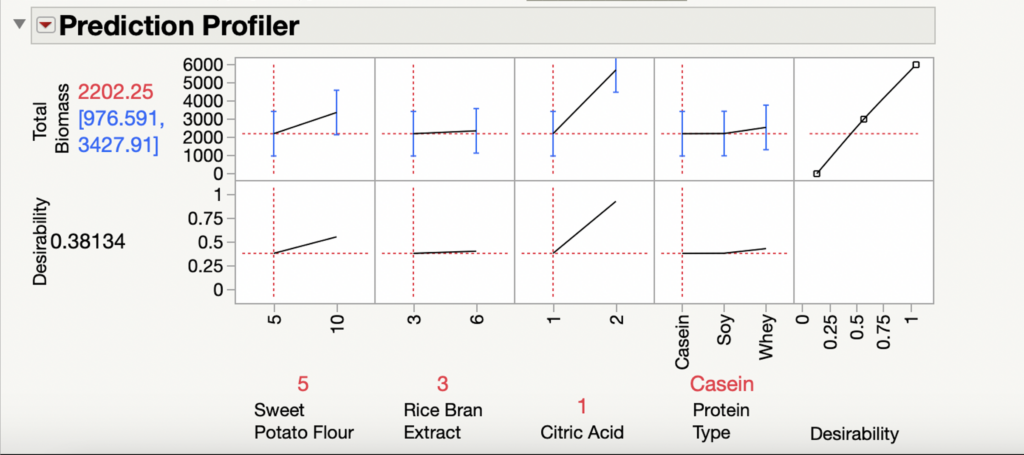
In Figure 2, the summary of the experiment is shown in terms of the total biomass that was derived from insects on the 24 different diets. This graphic representation helps us see clearly that the higher amount of sweet potato flour (10 g) gave superior results to the 5 g versions. The rice bran extract did not contribute substantially in either the 3 g or the 6 g versions. The higher level of citric acid (2 g) gave FAR better results than the lower (1 g). And the three proteins were not very different from one-another, with a possible trend towards the whey protein. What is not shown here (for reasons of simplicity) is that another graphic table in the JMP results shows which factors had interactions with other factors (positive or negative interactions). And this is one of the most exciting parts of using design of experiments with multi-factor components. It is, in my estimation, the interactions that really help explain how our rearing systems work.
By using a DOE approach we can better explore what factors are of significance in our rearing systems, the direction of their influence (positive or negative), and the factors which interact in ways that would not be intuitively predictable. Therefore, my teaching approach is to examine our rearing systems through the DOE approach! More about this will be presented in subsequent blogs and web pages.